Detecting the Unseen: Harnessing Anomaly Detection for Smart Building Data
- Yorgos Karafotias
- Jun 1, 2021
- 2 min read
Updated: Jun 1, 2023
Introduction:
Unlocking the full potential of IoT data in smart buildings has become paramount with the prevalence of IoT sensors generating a continuous stream of data. However, human supervision alone falls short in effectively exploiting this data due to its overwhelming volume. This is where the application of automated methods, specifically anomaly detection, becomes fascinating. Anomaly detection provides real-time analysis of time series data from smart building devices/sensors, enabling the identification of abnormal behaviors and potential problems.
Spotting the "Odd-One-Out": Exploring Time Series Anomalies in Smart Buildings:
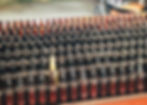
Within smart buildings, various types of time series anomalies pique our interest. We can examine individual sensor readings or entire sequences, as a subsequence may be suspicious even if its comprising values appear normal. Additionally, the analysis of sensor data in combination with contextual information, such as weather or calendar data, allows for the detection of more subtle anomalies. Furthermore, the ability to analyze individual sensors as separate data streams or combine them into multidimensional data streams captures the interplay between sensors, providing deeper insights.
Bandora's Quest for Robust Anomaly Detection:

At Bandora, our focus lies in developing robust anomaly detection (AD) solutions that can be readily applied to any smart building without the need for customization. To achieve this, AD must be source agnostic, adjustable, and adaptable. Sensor devices often generate radically different values in terms of range, magnitude, and regular operating modes. By automatically detecting a device's common operating modes, the interpretability of anomalies is enhanced. However, source agnosticism does not imply inflexibility. The ability to adjust the sensitivity of AD to suit specific monitoring requirements is a desirable characteristic. For example, allowing users to fine-tune the AD's sensitivity based on the importance of the monitored factors.
Adaptable AD: Embracing Change Without Manual Intervention:
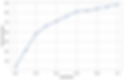
To ensure the longevity and effectiveness of anomaly detection systems, adaptability is crucial. Behaviors and devices within smart buildings are subject to change, and what was once considered anomalous may become the new norm. Repeated anomalous values may lose their significance over time, while outdated observations become irrelevant. User feedback plays a pivotal role in this aspect, as confirming a value as anomalous prevents the AD from becoming accustomed to it, maintaining its effectiveness in identifying anomalies.
Conclusion:
Harnessing the power of Anomaly Detection for Smart Building is key to unleashing the full potential of IoT data in smart buildings. By applying source agnostic, adjustable, and adaptable anomaly detection methods, we can effectively identify abnormal behaviors, detect potential problems, and take proactive measures to ensure optimal performance and safety. The continuous evolution and adaptability of anomaly detection systems equip smart buildings with the capability to embrace change, optimize efficiency, and pave the way for a smarter, more sustainable future.